Moneyball and Litigation: The Importance of a Standard Structure
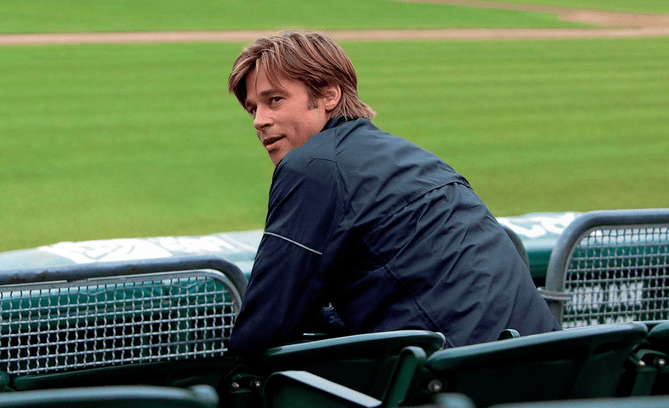
Unlocking the Power of Data in Litigation
Litigation has a data problem. Ask anyone who has ever looked at the existing litigation billing codes. One code is “analysis & strategy.” Of what? Of everything. That’s why this code alone accounts for a massive number of hours billed by outside litigation counsel.
The Data Dilemma in Litigation
Every year in-house counsel pay millions of dollars for this code. It infuriates them, but they have no other option. Outside counsel who enter these codes are equally frustrated. To them, it’s like being handed a wooden ruler with all the numbers sanded off and told to measure 3 ⅝ inches. So, they do their best and pick a code (often one that doesn’t really capture the work being delivered).
With this shaky foundation, the technology being built on top of it is just as shaky. The litigation market continues to layer new technologies on top of flawed data, to parse and repurpose it into marginally more useful constructs.
Understanding the Moneyball Revolution
Many litigation industry players are trying to be the Billy Beane of litigation, unlocking the Moneyball value of data that others miss. Moneyball analogies are frequent. In fact, a quick google search for “Moneyball and law” or “Moneyball and litigation” yields countless results.
So why hasn’t baseball’s Moneyball reality been realized by litigators? Why does Moneyball work for baseball but not litigation?
Released by author Michael Lewis in 2003, Moneyball profiled Billy Beane, General Manager of the Oakland A’s. Lewis showed how Beane and a team of data analysts in the back office of the Oakland A’s obsessively gathered and applied data. They sliced and diced data into untraditional statistics (focusing on “on base percentage” rather than the popular “slugging percentage” or “batting average”) to win far more Major League Baseball (“MLB”) games than they should have.
Indeed, with a third of the budget of other teams, like the New York Yankees, the Oakland A’s repeatedly reached the playoffs. Oakland had a team of players who looked underwhelming but consistently outperformed their opponents, time and again. Beane was able to spot talent that others missed because he was looking at data, not physical appearance or conventional wisdom.
The book and movie (starring Brad Pitt as Billy Beane) were wildly successful and remain relevant. And rightfully so. Moneyball captured the data revolution in baseball, showing how, for decades, MLB teams had been mismanaging and undervaluing baseball talent.
Baseball teams and their old-school scouts had been fixated on the physical appearances of players, not their performance on the field. The scouts used their own subjective assessments and “gut feelings.” They looked for “the good face” and how players behaved; this had gone on for decades.
Billy Beane exposed and corrected those mistakes. He dominated MLB by making player decisions and selecting draft picks by using cold data, ignoring physical appearances and gut feelings. In fact, he refused to watch the A’s play live because he knew it would cloud his objective thinking.
Beane silenced the biases of the scouts. Armed with data, Beane was able to cut through the noise and consistently identify baseball talent that others missed.
The Importance of Standardized Structure
As Lewis points out, the data revolution took off in baseball because of the explosion of player salaries. No longer could a team afford to blow money on a draft pick, with players being paid six- and seven-figure salaries. Sound familiar? Litigation has the exact same challenge, with outside litigation counsel hourly billing rates now climbing into the four-figure range.
Today, 20 years later, Moneyball has spread far beyond baseball. The data obsession of Moneyball permeates every sport, oftentimes down to the Little League or junior level. And within the legal industry, for those who want to make the case for using data, Moneyball is a popular citation.
Bad Data: The Elephant in the Room
On the surface, it seems impossible to argue against the point that more data results in better decisions, improved performance, and increased efficiency. The answer to the legal industry’s problems (and the problems of all other industries, too) is more data.
Or is it? What if the data is bad data? Or, as Lewis calls it in the book, “sloppy data.”
Not all data is equal. There is good (accurate and reliable) data and bad data. Data is not fungible, nor is it inherently accurate or reliable.
And if data is gathered using an inconsistent set of instruments, or if it is measured using a shifting series of metrics and benchmarks, should we be basing our decision making on it? Should we really run an industry that is worth hundreds of billions of dollars and determines the fates of companies and their employees using bad data?
No. But in our view, the advocates of applying Moneyball to litigation have missed this point. They have skipped first base in their haste to import data into the law, ignoring one major difference. Overlooked and taken for granted is that baseball has always had what litigation is missing: a standard and reliable structure.
The Need for Consistent Metrics in Litigation
Dating back to the mid-1800s, baseball is played using the same architecture every time: every game of baseball has nine innings, each inning has three outs, and each batter is allotted four balls and three strikes.
Baseball runs on a pure octane of baseball data because of this standard structure. This does not mean baseball statistical analysis is simple. Far from it. Moneyball’s most interesting parts (in our view) describe how baseball data analysis has evolved in the last few decades. Lewis explains how new statistics have been recognized as far more insightful than the popular “batting average” or “slugging percentage.” But that evolution was possible only because the structure of baseball didn’t vary when these new statistics were being gathered. It was possible because the standard structure of baseball stayed the same.
Baseball’s structure is super simple. Thousands of young children master the game of baseball every summer. And, yet, from this structure baseball analysts can draw mind-bending calculations and devise categories of statistics that are still being invented to this day. The individual, subjective whims of the players and coaches are allowed to surface in many ways, but they are never allowed to change the structure of the game.
Artificial Intelligence and the Future of Legal Analytics
And that, more than anything else, is why Moneyball is possible. That is why Moneyball works in baseball. If that structure were removed, baseball analytics would implode. Baseball statistics would quickly become just as unhelpful and incoherent as litigation billing records. Litigation has no such structure. This lack of structure is the root cause of several major gaps. It is the real reason why litigation training, case tracking, and time and billing are so inconsistent and shallow. The rules of civil procedure do not align with how litigation is practiced, and law schools do little to prepare new lawyers with the practical skills they need or any acclimation to the field of play. The benchmarks and metrics are always changing, and the billing codes do not match up with how litigators practice.
The outcome of all this is a mishmash of MacGyver-like devices and coping mechanisms that law firms, in-house clients, and individual lawyers use just to get by. There are no systems in place because systems require consistency; they require repeatable events.
Without any systems in place, barely keeping one’s head above water is seen as part of the job. It’s this struggle that, in part, makes litigation both a badge of honor for those who excel at it and the most derided legal practice for those that must pay for litigation. None of this is the fault of any individual litigation industry player. Nobody has offered a way to put numbers on the wooden ruler or to convert the yard stick into something that measures feet and inches, too. Nobody has offered a standard structure.
Charting a Path Forward: Embracing Structure
As a result, the incessant talk of artificial intelligence and data analytics is all puffery. It will remain an aspirational pitch without a standard structure. Bad data in equals bad data out. This is the elephant in the data room that everyone ignores, trying instead to graft solutions dependent on structure to our own unstructured industry.
Like other industries, litigation needs a structure upon which we can layer the tools to deliver, track, measure, and assess litigation training, management, billing, and, ultimately, outcomes. We need the equivalent of innings, outs, balls, and strikes from which we can make litigation repeatable, trackable, and meaningful.
Those litigators who are the first movers will become to litigation what Billy Beane was to baseball. Those who embrace this simple truth–that structure is required to make any activity meaningful–will discover profound insights, effective artificial intelligence, and actionable data analytics.
Conclusion: Moving Towards a Data-Driven Legal Industry
Baseball is a game. Litigation is anything but that. Both baseball and litigation have been prominent in America for hundreds of years. In 2020, it is long past time for the litigation industry to have a standard structure. The data revolution will remain aspirational so long as litigation continues to avoid solving this fatal flaw.
Article originally appeared in Texas Lawyer
Add Some Control to your Litigation with CONTROLit™
Discover how CONTROLit™ can streamline your process and eliminate junk draw litigation forever.
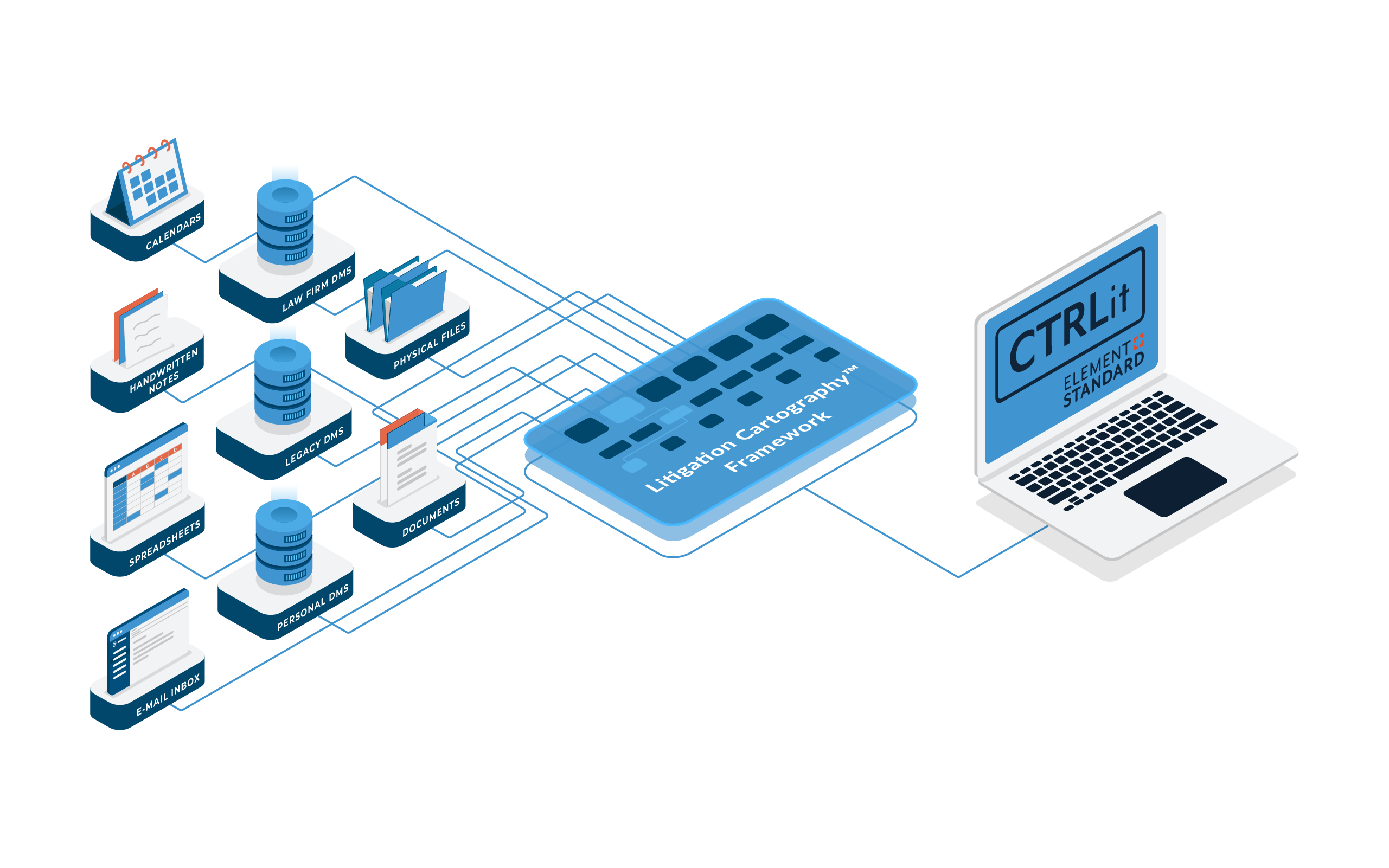